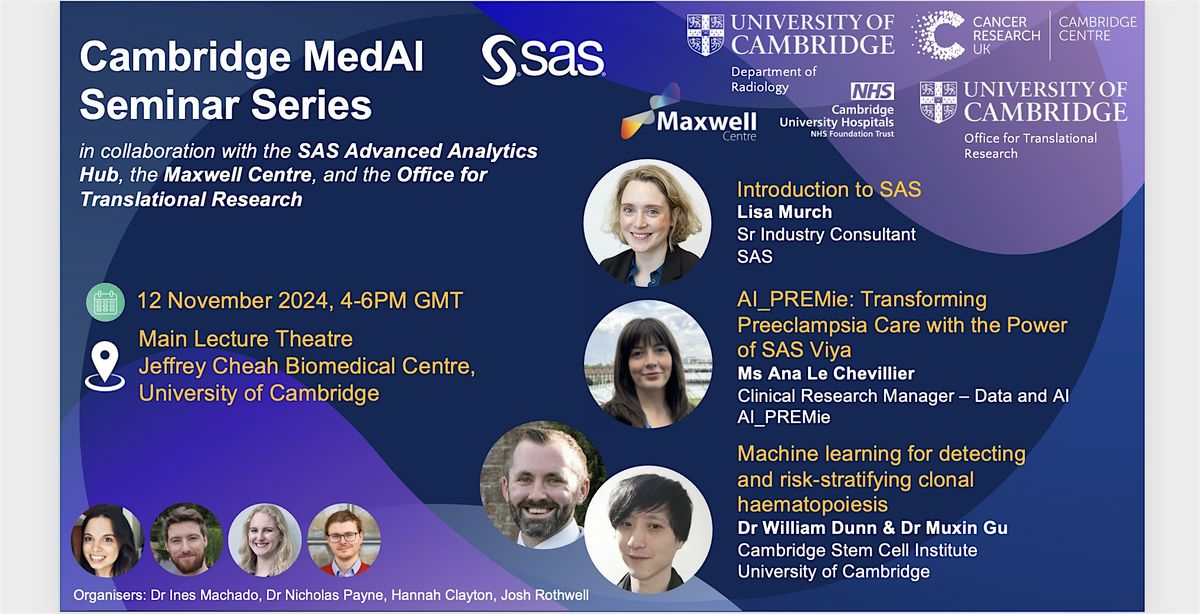
About this Event
Join us for the Cambridge AI in Medicine Seminar Series, hosted by the Cancer Research UK Cambridge Centre and the Department of Radiology at Addenbrooke's. This series brings together leading experts to explore cutting-edge AI applications in healthcare—from disease diagnosis to drug discovery. It's a unique opportunity for researchers, practitioners, and students to stay at the forefront of AI innovations and engage in discussions shaping the future of AI in healthcare.
This month's seminar will be a joint event with supported by , the and the . It follows the recent announcement of the “Cambridge and SAS launch partnership in AI and advanced analytics to accelerate innovation in the healthcare sector.”
The event will be held on 12 November 2024, 4-6pm at the Jeffrey Cheah Biomedical Centre (Main Lecture Theatre), University of Cambridge and streamed online via Zoom. This month will feature the following talks:
- Introduction to SAS: Lisa Murch, Sr Industry Consultant, Global Health and Life Sciences Customer Advisory, SAS
- AI_PREMie: Transforming Preeclampsia Care with the Power of SAS Viya: Ms Ana Le Chevillier, Manager of the AI Healthcare Hub at University College Dublin and Clinical Research Manager for Data and AI for AI_PREMie
- Machine learning for detecting and risk-stratifying clonal haematopoiesis: Dr William Dunn (CRUK Clinical Research Fellow) and Dr Muxin Gu (Research Associate), Cambridge Stem Cell Institute, University of Cambridge
This will be followed by a networking session over drinks and snacks, with a demo on the SAS Viya platform: Applying Machine Learning and Artificial Intelligence in Real World Data in Personalized Medicine for Non-Small Cell Lung Cancer Patients.
This is a hybrid event so you can also join via Zoom:
https://zoom.us/j/99050467573?pwd=UE5OdFdTSFdZeUtIcU1DbXpmdlNGZz09
Meeting ID: 990 5046 7573 and Passcode: 617729
-------------------------------------------------------------------------------------------
About SAS:
SAS delivers the most productive AI and analytics platform to transform data into life-changing insights and accelerate breakthroughs in life sciences. SAS aims to:
- Accelerate scientific innovation to bring therapies to market faster.
- Facilitate analytics decision-making and collaboration in a complex ecosystem.
- Evolve with a modern, open and flexible platform and industry solutions.
- Provide transparent and valid insights in a highly regulated environment.
-------------------------------------------------------------------------------------------
AI_PREMie: Transforming Preeclampsia Care with the Power of SAS Viya - Ms Ana Le Chevillier, Manager of the AI Healthcare Hub at University College Dublin and Clinical Research Manager for Data and AI for AI_PREMie
Ana Le Chevillier is Clinical Research Manager for Data and AI for AI_PREMie, a clinical decision support tool for preeclampsia, and Manager of the AI Healthcare Hub at University College Dublin. Ana leads the development of machine learning models for augmented clinical decision-making using SAS Viya. Her expertise extends to meticulous data quality and management across the entire data lifecycle, ensuring precision in healthcare analytics. Ana serves as an Ambassador for Woman in Data Science (WiDS) and was recently named in the Tech Top 30 under 30 list by the Business Post.
Abstract: Preeclampsia is a dangerous complication that can develop during pregnancy and causes the death of 76,000 mothers and 500,000 babies annually. Preeclampsia is characterised by the development of high blood pressure and protein in the urine. However, warning signs are difficult to detect, meaning that preeclampsia often goes undetected until complications become serious. Our team has drawn upon cutting-edge biomedical, clinical, and machine-learning research to develop a clinical decision support tool, AI_PREMie, that helps to identify individuals with preeclampsia and predict how they will progress. AI_PREMie has received multiple accolades including being rated as excellent in the UNESCO Global Top 100 AI projects addressing the 17 UN sustainable goals.
-------------------------------------------------------------------------------------------
Machine learning for detecting and risk-stratifying clonal haematopoiesis – Dr William Dunn (CRUK Clinical Research Fellow) and Dr Muxin Gu (Research Associate), Cambridge Stem Cell Institute, University of Cambridge
Billy is a clinical haematologist studying for a PhD co-supervised by Prof George Vassiliou (clinician-scientist) and Dr Irina Mohorianu (Turing Fellow in Machine Learning). He completed his undergraduate medical training at the University of Glasgow, followed by a Masters in Bioinformatics, before moving to Cambridge to commence an Academic Clinical Fellowship. His main interests are in applying machine learning and data science approaches to detect and characterise clonal haematopoiesis.
Muxin is a computational biologist specialised in genomics. He is currently in Dr George Vassiliou’s group at Cambridge Stem Cell Institute, analysing large datasets of genomic data. Muxin’s current research interest is to investigate the stepwise change of genomic landscape prior to the onset of cancer and to understand the evolutionary trajectory of cancer development.
Abstract: Clonal haematopoiesis (CH), the disproportionate expansion of a haematopoietic stem cell and its progeny, driven by somatic gene mutations, is a common age-related phenomenon that engenders an increased risk of developing myeloid neoplasms. At present, CH is identified by targeted sequencing of peripheral blood DNA, which is impractical to apply at population scale. The complete blood count (CBC) is an inexpensive, widely used clinical test. Here, we explore whether tree-based machine learning approaches applied to CBC data can identify individuals likely to harbour CH and prioritise them for DNA sequencing. We showcase a proof-of-concept that the presence of high-risk CH can be inferred from CBC perturbations using machine learning classifiers, and identify challenges in implementing this as a screening test.
Using whole-exome sequencing data from 454,340 UK Biobank participants, of whom 1808 developed myeloid caner 0-15 years after recruitment, we compared genetic, haematological and biochemical profiles between the pre-cancer and control groups, finding that disease-specific changes are detectable years before diagnosis. Using these, we construct MN-predict (https://bioinf.stemcells.cam.ac.uk/shiny/vassiliou/MN_predict/), a web application that generates time-dependent predictions of myeloid cancer with input of basic blood test and genetic data. Our study demonstrates that individuals that develop myeloid cancer can be identified years in advance and provides a framework for disease-specific prognostication that will be of substantial use to researchers and physicians.
-------------------------------------------------------------------------------------------
We look forward to your participation! If you are interested in getting involved and presenting your work, please email Ines Machado at [email protected]
For more information about this seminar series, see: https://www.integratedcancermedicine.org/research/cambridge-medai-seminar-series/
Event Venue & Nearby Stays
Jeffrey Cheah Biomedical Centre, Puddicombe Way, Cambridge, United Kingdom
GBP 0.00